Using machine learning and big data to detect fraud is common in numerous industries, including healthcare, finance, and retail, and it needs to be central to your marketing fraud detection plan.
Three Key Techniques for Fraud Detection
In this blog, we’re focusing on three key techniques that your fraud protection partner should be able to perform: browser-side analysis, server-side analysis, and data mining.
1. Browser-side Analysis
Browser-side analysis looks at the remnant clues around the browser session that convincingly, but imperfectly, mimic legitimate traffic. Among the attributes examined, this analysis will look for:
- Traces of malware
- Anomalous on-page behavior
- Faked domains and app ids
- Deceptive placement handling by malicious publishers and mobile botnets
Browser-side analysis can be used to detect many types of placement fraud committed by malicious publishers or domain spoofing, app spoofing, and device emulation perpetrated by mobile botnets.
2. Server-side Analysis
By collecting client data such as IP address, ISP info, and so on, server-side analysis is able to compare this data to a variety of fraud databases that are built off of historical fraud activity. Server-side analysis conforms with the GIVT (general invalid traffic) guidelines and effectively identifies invalid traffic through list-based filtration mechanisms. GIVT detection techniques can, for instance, use IP lookups to identify suspicious utilization of proxy servers and hosting providers—a deployment technique that is commonly used by botnet operators.
3. Data Mining
Unsupervised machine learning can produce clusters of “like” traffic. Supervised machine learning can determine which of those clusters are fraud (exposing marginal lookalike instances of fraud) and feed this data back into heuristics, exposing fraudulent activity that may have been missed by the initial sweep of browser-side or server-side fraud analysis. Data mining techniques can be used to surface anomalous patterns that are found in automated botnet traffic, device farms, emulators and other perfidious fraud tactics. As always, any machine learning algorithm works best when monitored, trained, calibrated, and overseen by data scientists with in-depth fraud expertise.
How These Big Data and Machine Learning Amplify Fraud Detection
What’s particularly powerful about leveraging these three techniques is that they circuitously inform each other to enrich and amplify fraud detection. Consider the following schematic.
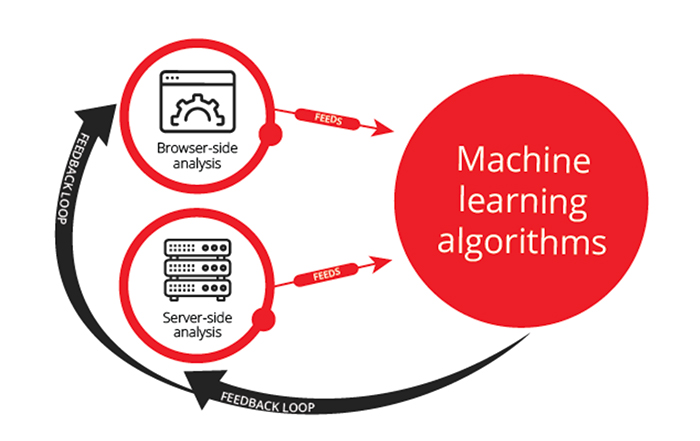
Not only do machine-learning algorithms “learn” from discoveries from browser-side and server-side analysis, but their results can also be used to identify other characteristics of fraudulent traffic that can be flagged on the browser-side and server-side analysis, creating a virtuous loop.
Remember, you want to verify that the fraud detection vendor you select employs all three methods to ensure optimal fraud detection. How can you tell if your verification partner is performing all the right actions to keep campaigns accurate and true? Download our guide, 14 Things to Ask When Looking for a Verification Partner, that provides answers to the vital questions you need to ask!